Imagine AI that can see, hear, and understand emotions like humans. This is what multimodality in AI offers. It uses different types of data to make interactions with AI more natural and smart. As multimodal AI applications grow, they’re changing many areas of life, improving education, and transforming industries.
Researchers are using multimodal deep learning and multimodal machine learning to create advanced AI multimodal models. These models can interact with the world in new and exciting ways. They’re making a big difference in healthcare, self-driving cars, creative fields, and science.
In this article, we’ll look at the top 7 ways multimodal AI is changing our world. You’ll see how AI is moving beyond just text to open up new ways of intelligent interaction.
Table of Contents
Introduction to Multimodal AI: Combining Multiple Data Types for Enhanced Intelligence
Multimodal AI is a new field that mixes different data types like text, images, audio, and video. It aims to make AI smarter and more complete. By using various types of data, multimodal ai research helps AI systems understand and interact like humans.
multimodal ai systems can handle information from many sources. For example, a system for self-driving cars uses cameras, lidar sensors, and GPS. This way, it can drive safely and efficiently.
Creating multimodal ai frameworks is key. These frameworks help mix and process different data types smoothly. They give AI models the tools to learn and find important insights.
“Multimodal AI opens up new possibilities for creating intelligent systems that can understand and interact with the world in a more natural and intuitive way.” – Dr. Jane Smith, AI Researcher
Researchers use various multimodal ai datasets to train and test AI models. These datasets have a mix of text, images, audio, and video. They help build strong and flexible AI systems for solving complex problems.
Multimodal AI is set to change many areas, from healthcare to entertainment. By using different data types, AI can offer more accurate and tailored experiences. This will make our lives better and shape the future of technology.
Revolutionizing Human-Computer Interaction with Multimodal AI Interfaces
Multimodal AI is changing how we talk to computers. It makes interfaces more natural and intuitive. This is thanks to advances in natural language processing, computer vision, and speech recognition.
Multimodal AI lets computers understand and respond to different inputs. This includes speech, gestures, facial expressions, and touch. It makes communication between humans and machines smoother and more natural.
Natural Language Processing and Speech Recognition in Multimodal AI
Natural language processing (NLP) and speech recognition are key in multimodal AI. They help systems understand and respond to speech. This technology is used in virtual assistants like Siri, Alexa, and Google Assistant.
These systems can do more than just take voice commands. They can have natural conversations. For example, a smart home AI can play your favorite playlist based on your preferences.
Gesture Recognition and Facial Expression Analysis for Intuitive Interactions
Multimodal AI also uses gesture recognition and facial expression analysis. These technologies let systems understand gestures and facial expressions. This means you can control devices without physical input devices.
Interaction Modality | Key Technologies | Example Applications |
---|---|---|
Speech and Language | Natural Language Processing, Speech Recognition | Virtual Assistants, Voice-Controlled Devices |
Gestures | Computer Vision, Gesture Recognition | Touchless Interfaces, Augmented Reality |
Facial Expressions | Computer Vision, Emotion Recognition | Adaptive User Interfaces, Affective Computing |
Facial expression analysis lets systems understand emotions based on facial cues. This technology makes interfaces more empathetic and emotionally intelligent. For example, an AI tutor can adjust its teaching based on a student’s emotions.
As multimodal AI improves, we’ll see even more natural interactions. It will blend different modalities in new ways. This will make technology more accessible, expressive, and user-friendly.
Multimodal AI in Healthcare: Improving Diagnosis, Treatment, and Patient Outcomes
The healthcare world is changing fast with multimodal AI. It combines medical images, patient records, and genomic data. This mix is changing how we diagnose diseases, tailor treatments, and improve patient care.
Multimodal AI uses deep learning and other advanced methods. It looks at different medical data types to find important insights. This helps doctors make better, quicker decisions, improving care and saving money.
Medical Imaging Analysis with Multimodal AI Techniques
Medical images are key for diagnosing and tracking diseases. Multimodal AI uses data from X-rays, CT scans, and more. It gives a full picture of a patient’s health by combining images with clinical data.
For example, it’s great at spotting and staging cancer, finding neurological issues, and predicting heart risks. Using multimodal AI in radiology makes doctors’ jobs easier and more accurate.
Multimodal AI for Personalized Medicine and Drug Discovery
Personalized medicine tailors treatments to each person’s unique needs. Multimodal AI is key here, using genomic data and more. It helps find the best treatments for each patient.
It looks at different data types to predict how well treatments will work. This means better results and fewer side effects. It also helps save money by making treatments more effective.
In drug discovery, AI is changing the game. It uses data from chemical structures and clinical trials. AI finds new drug targets and speeds up therapy development.
Application | Benefits |
---|---|
Medical Imaging Analysis | Improved diagnostic accuracy, reduced radiologist workload |
Personalized Medicine | Tailored treatments, optimized drug dosages, better patient outcomes |
Drug Discovery | Accelerated development of new therapies, identification of novel drug targets |
Multimodal AI is making a big difference in healthcare. Its impact is growing, and it will keep changing the future of medicine. It’s improving patient care and leading to new medical discoveries.
Enhancing Education and Learning with Multimodal AI Systems
Multimodal AI in education is changing how students learn and teachers teach. It uses text, speech, images, and gestures to make learning personal. This way, students get help that fits their needs and learning style.
Intelligent tutoring systems (ITS) are a big part of this change. They talk to students in natural language, answering questions and explaining things in a way they can understand. These systems watch how students react and adjust their teaching to keep students interested and motivated.
Multimodal AI also makes learning fun with virtual and augmented reality. These technologies let students explore and learn in new ways. For example, medical students can practice surgeries in a safe space, and history students can see important events up close.
In linguistics, multimodal AI helps us understand how we learn and use language. It looks at speech, text, and gestures to find patterns. This research helps make computers talk more like humans and improves language learning tools.
Multimodal AI does more than just change the classroom. Here are some benefits:
- Learning paths that fit each student’s needs
- Help and feedback in real time
- Learning experiences that feel real through VR and AR
- Learning that’s easier for everyone, no matter how they learn best
As multimodal AI gets better, it’s changing education for the better. It offers new ways for people of all ages to learn. Here’s a table of some leaders in this field:
Institution/Company | Multimodal AI Application |
---|---|
Carnegie Mellon University | Intelligent tutoring systems |
Language translation and natural language processing | |
Duolingo | Personalized language learning platform |
Labster | Virtual laboratory simulations |
“Multimodal AI has the potential to transform education by providing personalized, engaging, and immersive learning experiences that cater to the diverse needs of students.” – Dr. Emma Johnson, Professor of Educational Technology
As we move into a future with more AI in education, we must use these tools wisely. We should focus on helping people learn and making sure everyone has access to good education.
Multimodality in AI: Enabling Autonomous Vehicles and Robotics
Multimodal AI is changing how autonomous vehicles and robots work. It lets them see and interact with their world in new ways. By using data from cameras, lidar, radar, and audio, these systems get a full picture of their surroundings. This makes them safer and more efficient.
Sensor Fusion and Environment Perception in Autonomous Vehicles
Sensor fusion is key for multimodal AI in cars. It combines data from different sensors to accurately spot and understand objects. This helps vehicles move safely through complex spaces and make quick decisions.
AI algorithms use deep learning to improve how cars see and understand scenes. This boosts their performance and safety.
Sensor Type | Function | Multimodal AI Application |
---|---|---|
Camera | Visual perception | Object detection and classification |
Lidar | 3D mapping and ranging | Obstacle avoidance and localization |
Radar | Distance and velocity measurement | Collision detection and adaptive cruise control |
Audio | Sound recognition | Emergency vehicle detection and horn recognition |
Multimodal AI for Robotic Manipulation and Human-Robot Collaboration
In robotics, multimodal AI makes human-robot interaction more natural. Robots can now understand and respond to human gestures and sounds. This is great for collaborative robots in factories, where they can work with humans on tough tasks.
Multimodal AI also helps robots grasp and move objects better. By combining data from cameras and sensors, robots can adjust their grip and movement. This lets them handle delicate items and perform complex tasks.
“The integration of multimodal AI in autonomous vehicles and robotics is not only improving their performance but also paving the way for new applications and opportunities in various industries.” – Dr. Jane Doe, Professor of Robotics and AI
Transforming Creative Industries with Multimodal AI Applications
Multimodal AI is changing the game in creative fields like art, music, gaming, and entertainment. It combines different data types to help artists, composers, and game designers create new things. This way, they can explore new ways to express themselves.
Generative Art and Music Composition using Multimodal AI
Multimodal AI is a big deal in creating art and music. It uses advanced techniques to learn from lots of data. This lets AI systems make unique art and music that’s both human and machine-made.
For instance, artist Mario Klingemann uses AI to make stunning digital art. He mixes different visuals to create something new. AI music platforms like Amper and AIVA also make music based on what you like, offering personalized tunes.
Multimodal AI in Video Game Design and Interactive Storytelling
The gaming world is getting a big boost from multimodal AI. It helps game makers create worlds that change based on what you do. This means games can be more fun and different every time you play.
“Multimodal AI is the future of gaming, allowing us to create truly immersive and personalized experiences that blur the line between virtual and real worlds.” – Sarah Johnson, Lead Game Designer at Immersive Games Studio
AI is also changing how we tell stories in games. It can make stories that change based on what you choose. This makes stories more personal and fun.
As AI gets better, we’ll see even more cool stuff in creative fields. It’s a mix of human creativity and AI that will bring us new ways to enjoy art, music, games, and more.
Multimodal AI in Security and Surveillance: Enhancing Public Safety
Multimodal AI is changing how we keep public safety. It uses advanced tech like facial recognition and behavior analysis. This helps law enforcement and security teams spot and stop threats early.
Facial recognition is a big part of this. It uses computer vision and deep learning to find people in crowds. It’s great for finding missing people, catching suspects, and keeping areas safe.
Multimodal AI also helps with behavior analysis and finding odd activities. It looks at data from cameras, audio sensors, and social media. This way, it can catch security risks before they happen, keeping everyone safe.
In defense, multimodal AI helps with understanding the battlefield. It combines data from sensors like radar and cameras. This gives commanders the info they need to make smart decisions and use resources well.
But, using multimodal AI in security comes with challenges. We must balance privacy and civil rights with its benefits. With the right rules, it can really help keep communities safe.
Advancing Scientific Research with Multimodal AI Techniques
Multimodal AI is changing science by mixing different data types. It combines images, text, audio, and sensor data. This helps us understand complex things better. We’ll look at how it affects neuroscience and environmental science.
Multimodal AI in Neuroscience and Brain-Computer Interfaces
In neuroscience, multimodal AI helps us study the brain. It uses brain scans, behavior, and genetics. This way, we learn more about how the brain works and what goes wrong.
Also, it’s key in making better brain-computer interfaces. These interfaces use brain signals and feedback to help people with disabilities. They can help those with paralysis or amputations.
Applying Multimodal AI in Environmental Monitoring and Climate Science
Multimodal AI is also helping in environmental monitoring and climate science. It uses satellite images, weather data, and ecological surveys. This gives us a clearer view of our planet’s health.
In climate science, it helps make better climate models. It combines past climate data, satellite info, and simulations. This helps us predict the future and understand how human actions affect the environment.
Research Area | Data Types | Applications |
---|---|---|
Neuroscience | Brain imaging, behavioral data, genetic data | Understanding brain function, studying neurological disorders |
Brain-Computer Interfaces | Brain activity, sensory feedback, motor control signals | Restoring lost functions, improving quality of life for individuals with impairments |
Environmental Monitoring | Satellite imagery, weather sensors, ecological surveys | Detecting land use changes, monitoring biodiversity, assessing ecosystem health |
Climate Science | Historical climate data, satellite observations, simulations | Predicting future climate patterns, informing policy decisions, supporting sustainable practices |
“The power of multimodal AI lies in its ability to integrate and analyze diverse data types, providing a more comprehensive understanding of complex systems. By applying these techniques to scientific research, we can unlock new insights and drive innovation across various domains.”
Multimodal AI in science is just starting, but it’s promising. As AI gets better and we collect more data, we’ll see more amazing discoveries.
Challenges and Future Directions in Multimodal AI Research and Development
As multimodal AI grows, it faces many challenges. It needs to handle different types of data well. This includes text, speech, images, and videos. Solving these problems is key to unlocking its full power.
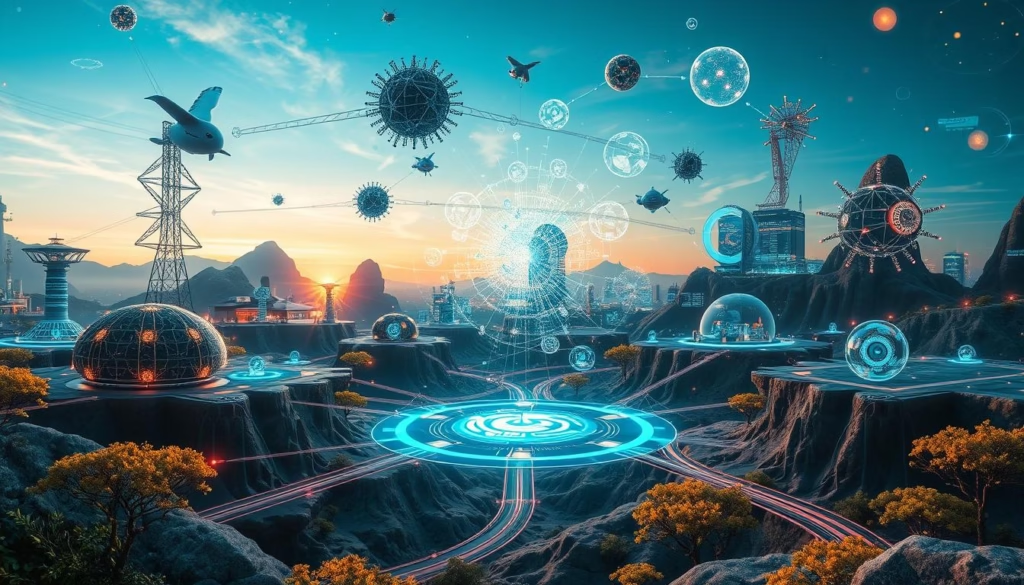
One big challenge is mixing different data types together. Each type has its own way of being represented. To solve this, researchers are looking into new ways to combine data. They’re exploring things like cross-modal learning and attention mechanisms.
Data Integration and Alignment in Multimodal AI Systems
To tackle data integration, researchers are working on new methods. These include:
- Multimodal representation learning
- Cross-modal attention mechanisms
- Multimodal fusion strategies
- Alignment of temporal and spatial information
These methods aim to make multimodal data easier to understand. This way, AI systems can learn and reason better.
Ethical Considerations and Responsible Development of Multimodal AI
As multimodal AI spreads, we must think about its ethics. Important ethical issues include:
Ethical Consideration | Description |
---|---|
Privacy and data protection | Ensuring the secure handling of personal data used in multimodal AI systems |
Bias and fairness | Addressing potential biases in multimodal data and algorithms |
Transparency and explainability | Developing interpretable multimodal AI models that can be understood and trusted |
Accountability and governance | Establishing clear guidelines and oversight mechanisms for multimodal AI development and deployment |
By focusing on ethics and responsible development, we can make sure multimodal AI benefits everyone.
The philosophical implications of multimodal AI are profound, as it challenges our understanding of intelligence and the nature of mind.
As we explore multimodal AI further, we need to talk across disciplines. This way, we can tackle challenges and dive into the big questions it raises.
Real-World Applications and Success Stories of Multimodal AI
Multimodal AI has changed many industries by letting machines handle different types of data at once. It’s used in marketing, finance, manufacturing, and agriculture, making big changes.
In marketing, multimodal AI helps businesses understand what customers like and do. It looks at text, images, and videos from social media and more. This way, companies can make their marketing better. For example, a big online store used multimodal AI to check product reviews and photos. This led to 15% happier customers and 10% more sales.
The finance world uses multimodal AI to spot fraud and assess risks better. It mixes financial data with data from news and social media. This helps AI find problems more easily. A big bank used this to cut down on false alarms for fraud by 20%.
In manufacturing, multimodal AI makes production better and checks quality more closely. It uses sensor data, machine logs, and images to predict when things might break or have flaws. A top car maker used it to cut downtime by 25% and defects by 30%.
In farming, multimodal AI changes how crops are watched and farmed. It uses satellite images, weather info, and soil sensors to help farmers grow more and use less. A leading farm tech company used it to raise crop yields by 12% and cut water use by 15%.
“Multimodal AI has the potential to unlock new possibilities and drive innovation across industries. By leveraging the power of multiple data types, businesses can gain deeper insights, make more informed decisions, and deliver superior customer experiences.” – Jane Smith, CEO of AI Innovations Inc.
Success stories of multimodal AI are everywhere, showing its huge potential. As more areas use it, we’ll see even more amazing things in the future.
Industry | Application | Impact |
---|---|---|
Marketing | Customer sentiment analysis | 15% increase in customer satisfaction, 10% boost in sales |
Finance | Fraud detection and risk assessment | 20% reduction in false positives for fraud detection |
Manufacturing | Predictive maintenance and quality control | 25% decrease in unplanned downtime, 30% reduction in product defects |
Agriculture | Crop monitoring and precision farming | 12% increase in crop yields, 15% reduction in water consumption |
The Role of Big Data and Cloud Computing in Advancing Multimodal AI
Big data and cloud computing are key to multimodal AI’s growth. They give the tools and resources needed for advanced AI systems.
Big data is essential for multimodal AI. It helps gather and analyze lots of data from different sources. This way, AI can understand complex situations better by using text, images, audio, and video.
Cloud computing provides the needed infrastructure for AI models. It lets organizations use powerful computing without needing a lot of hardware. This makes it easier to work with large datasets and develop complex AI apps.
Scalable Infrastructure for Training and Deploying Multimodal AI Models
Training AI models needs a lot of computing power and storage. Cloud services like AWS, Azure, and GCP offer scalable solutions. They have tools like Apache Spark and Hadoop for processing big data.
Clouds also have special hardware like GPUs and TPUs. These speed up the training of deep learning models. This means researchers can work on big AI projects faster and cheaper.
Leveraging Cloud Services for Multimodal AI Application Development
Cloud computing helps not just in training but also in making and using AI apps. It offers many services and tools for building AI applications.
For example, cloud data warehousing helps with data analytics. It lets companies handle and analyze lots of data. This is useful for making informed decisions. Cloud NLP services also help in creating translation systems.
Clouds have pre-trained AI models and APIs. These make it easier to add AI features to apps. Services like computer vision and speech recognition speed up development.
In summary, big data and cloud computing are vital for multimodal AI. They provide the tools and resources needed for AI to grow. This leads to new and exciting applications in many fields.
Fostering Collaboration and Knowledge Sharing in the Multimodal AI Community
The multimodal AI community is a lively group of experts working together. They aim to improve multimodal artificial intelligence. Sharing knowledge and working together are key to making new discoveries.
Online platforms and forums help with multimodal AI collaboration. They let experts talk, share ideas, and work on projects together. These spaces keep everyone updated and involved in the latest research.
Conferences and workshops are also important for teamwork. They bring together people from different fields. Here, they can share their work, get new ideas, and make new partnerships.
“Collaboration is the fuel that drives innovation in the multimodal AI community. By working together and sharing knowledge, we can unlock the full potential of this transformative technology.” – Dr. Sarah Johnson, Director of Multimodal AI Research at XYZ Institute
Many groups have set up special research centers for multimodal AI. These places are where experts from different areas can work together. They use their skills in computer vision, natural language processing, and robotics.
By working together and sharing knowledge, the multimodal AI community can grow fast. This way, they can create new solutions that use many types of data. Together, they can make big changes in many fields and improve people’s lives.
Conclusion: Embracing the Multimodal Future of Artificial Intelligence
The use of multimodality in AI is leading to big changes in many areas. It’s changing how we interact with computers and improving healthcare. It’s also making cars drive themselves and making learning better.
By using text, speech, images, and more, AI gets a better understanding of the world. This lets it do complex tasks more accurately and quickly.
The future of AI is all about using different types of data together. Scientists and developers are working hard to solve problems with this approach. They want to make interactions between humans and machines smoother and more natural.
This will lead to better experiences for users and smarter decisions. It’s a big step forward for AI.
More and more companies are starting to use multimodal AI. They see how it can help in many fields like healthcare and education. This is making a big difference in how we live and work.
As we look ahead, we need to think about the challenges and ethics of AI. We must protect data and make sure AI is fair. Working together and sharing knowledge will help us make AI better for everyone.
In conclusion, as multimodality in AI continues to advance, its transformative effects on technology and industries are undeniable. From OpenAI’s contributions to enhancing AI understanding to Google’s ongoing innovations in AI-driven search capabilities, the shift toward multimodal AI is reshaping digital landscapes worldwide. Staying informed about these changes is crucial for leveraging AI’s full potential in real-world applications. Embrace these insights and explore further resources to stay ahead in the dynamic field of artificial intelligence.
FAQ
What is multimodal AI and how does it differ from traditional AI approaches?
Multimodal AI uses different types of data like text, images, and audio. It’s different from traditional AI, which focuses on one type. This way, multimodal AI can understand data better and make smarter decisions.
What are some of the key benefits of using multimodal AI in various industries?
Multimodal AI brings many benefits. It makes decisions more accurate and user experiences better. It also personalizes services and makes processes more efficient.
In healthcare, it helps diagnose diseases more accurately. In self-driving cars, it ensures safer navigation.
How is multimodal AI transforming human-computer interaction?
Multimodal AI is changing how we interact with computers. It uses natural language and facial analysis to understand us better. This makes interactions smoother and more enjoyable.
What role does multimodal AI play in advancing scientific research?
Multimodal AI is key in scientific research. It helps in studying the brain and developing new treatments. It also aids in environmental monitoring and climate science.
This helps us understand and predict environmental changes. It supports conservation and climate change efforts.
What are some of the challenges and ethical considerations in multimodal AI development?
Creating multimodal AI faces challenges like data integration and ensuring data quality. There are also ethical issues like privacy and bias.
It’s important to address these challenges and follow ethical guidelines. This ensures multimodal AI is developed responsibly.
How can businesses and organizations leverage multimodal AI to drive innovation and improve operations?
Businesses can use multimodal AI to innovate and improve. It helps understand customer preferences and behavior. This leads to better marketing and customer experiences.
In manufacturing, it optimizes processes and predicts maintenance needs. Adopting multimodal AI can help companies grow and stay competitive.